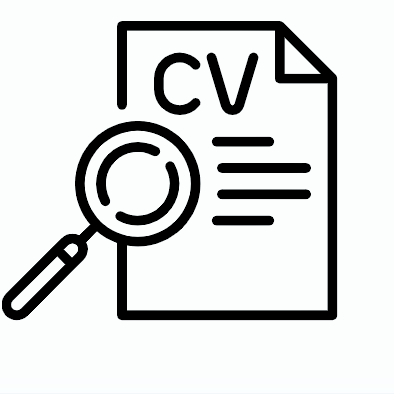
Mirjana Kljajić Borštnar received her Ph.D. in Management Information Systems from the University of Maribor. She works as an Associate Professor at the Faculty of Organizational Sciences, University of Maribor and is a member of Laboratory for Decision Processes and Knowledge-Based Systems. She has been involved in many EU and national projects. Her research work covers data-driven decision support systems, data mining, multi-criteria decision-making, and organizational learning. She co-authored several scientific articles published in recognized international journals, including Expert Systems with Application, PLOS ONE, Industrial Management & Data Systems, Group Decision and Negotiation and others. She is an editorial board member of Uporabna informatika journal, program committee member of Bled eConference, DataScience conference, International Symposium on Operations Research in Slovenia, and research representative in national Strategic Research & Innovation Partnership Smart Cities and Communities for Artificial Intelligence.
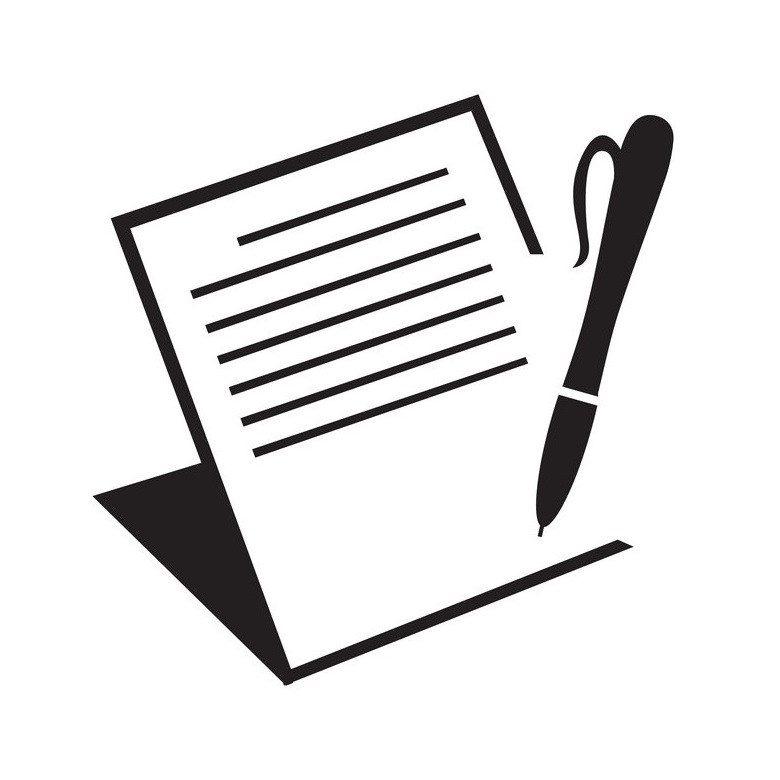
The importance of understanding machine learning models in an organizational setting
Decision making in an organizational setting is a complex process involving a group of people from interdisciplinary fields and in different roles. In the "data-driven" paradigm (from data-driven organization to data-driven innovation to data-driven decision making), it is suggested that data can add value to the organization. However, in many cases we have observed that this is not the case. Data Analysts and Data Scientists are not the only ones who can turn data into value. It is the understanding of complex organizational processes and ill-defined problems that, along with sophisticated data analytics, can transform data into business value and advantage. We derive our thesis from the idea that complex organizational problems can be efficiently supported by data analytics if the following conditions are met: 1) data analytics is driven by well-defined problems - the right questions are asked, 2) we have high quality data, and 3) data-driven models are well understood and thus support organizational learning. To illustrate our assumptions, we will present three real-world use cases of machine learning model implementation: 1) business-to-business sales prediction, 2) terminal call rate prediction during warranty period, and case 3) classification of older electronic texts in Universal Decimal Classification.
Keywords: data-driven decision support, organizational learning, user acceptance, machine learning, explanations