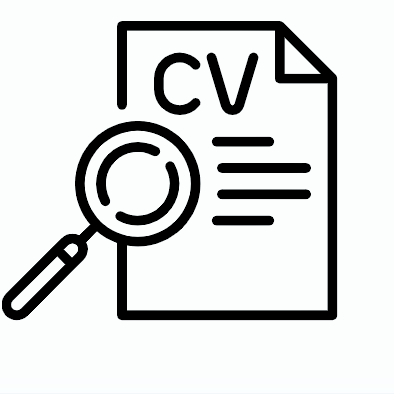
Born in 1951, Georg Pflug studied Law, Mathematics and Statistics at the University of Vienna. He was Professor at the University of Giessen, Germany and is Full Professor emeritus at the Faculty of Business, Economics and Statistics of the University of Vienna He is also part time research scholar at the International Institute of Applied Systems Analysis, Laxenburg, Austria. Georg Pflug's interests include Stochastic Modeling, Stochastic Optimization, Measuring and Managing of Risks and Applications in Finance (including Pension Funds), Insurance, Energy and Shape Design. Georg Pflug held visiting positions University of Bayreuth, Michigan State University, University of California at Davis, Université de Rennes, Technion Haifa and Princeton University. He is currently Associate Editor of 10 international journals and author of 5 books, editor of 6 books, and more than 160 publications in refereed journals.
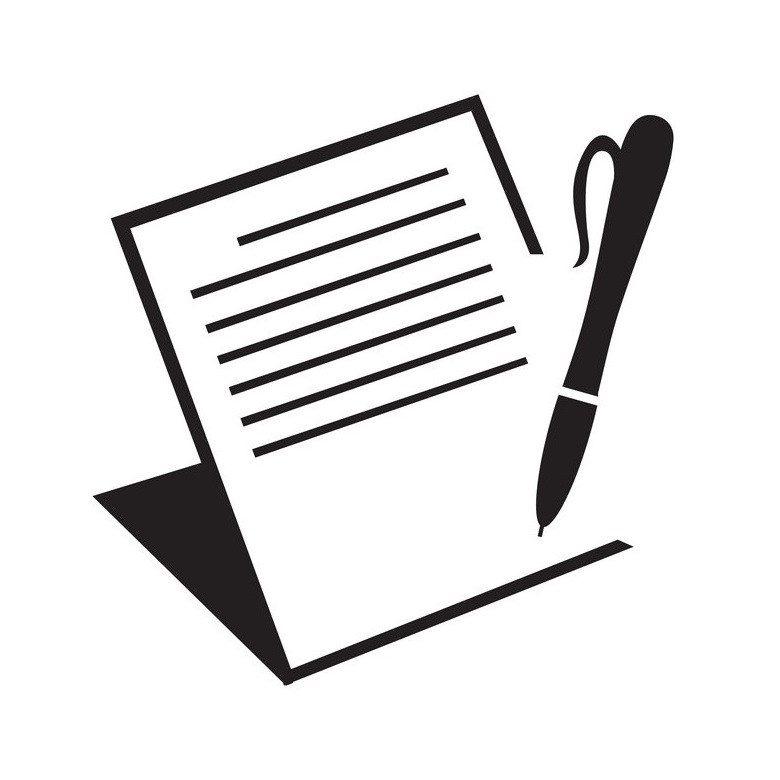
Financial Optimization: From data to models to solutions
Decision making in Quantitative Finance requires to establish a mathematical model for the considered financial processes. Very often, the model class is chosen ad hoc and without reference to the data; only as a second step data are fitted to the chosen model class. To put it differently, the modeler chooses the framework out of his/her imagination and the empirical check comes later. This reminds to the saying: If you have a hammer, everything looks like a nail. In this talk, we take a different viewpoint. On one hand, (1) we introduce parameter free methods of estimation of discrete stochastic processes for financial optimization and on the other hand (2) we discuss the notion of distributionally robust decision making, that is decision making, when there is some uncertainty about the correct model.
The main structure to be investigated is a stochastic tree, i.e. a discrete stochastic process. Such trees can be considered as approximations to real data processes. In topic (1) we show how to minimize the distance between observation data and the model by some learning strategies. In topic (2) we consider a set of several models, which are all compatible with the observed data and choose a decision which is robust w.r.t. model error. In modeling, one typically cannot avoid to make some assumptions. However, we argue that the aim should be to use as much as possible empirical information in order to avoid a modeler bias. Our approaches will be illustrated by concrete examples.